(RA-L 2024) EnKode - Active learning of flows with Koopman operators
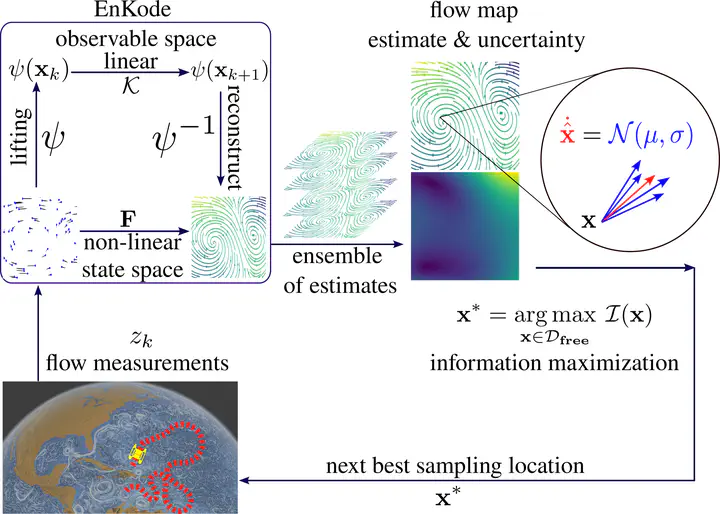
Koopman operator theory can be used to provide a functional form of the flow of nonlinear dynamical systems, as well as physically interpretable feature extraction in the form of coherent sets. Both are valuable for downstream robotics tasks such as path planning.
However, collecting data to build these models is expensive. Therefore, in this work, we propose EnKode, a method based on Koopman theory and ensemble methods that models flows and provides an active sensing strategy to guide robots to collect sparse informative data for the flow model. From our knowledge, it is the first works to apply Koopman theory to the task of robotic active sensing and modeling of flows.
In evaluating our methods on three flows of increasing complexities, including real ocean flows, we show that EnKode provides high quality uncertainty quantification in comparison to a Gaussian Process regression model baseline; multi-scale modeling capabilities; and an effective active sensing strategy.
Relevant Publication:
Li, Alice K., Thales C. Silva, and M. Ani Hsieh. “EnKode: Active Learning of Unknown Flows with Koopman Operators.” IEEE Robotics and Automation Letters (2024). Link to paper.